What Is a Data Analytics Framework, And What Types Of Data Analytics Exist?
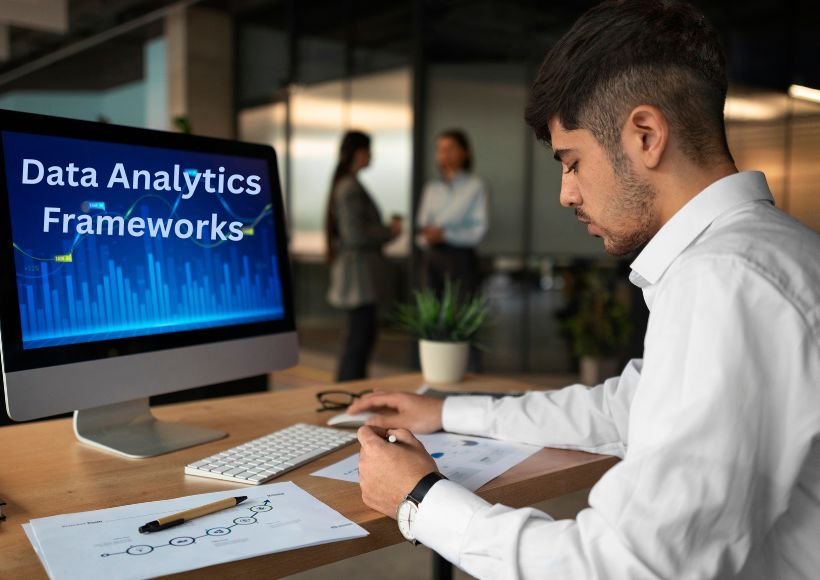
Modern business strategies rely on data. But it takes more than simply having access to this information: you must know how to utilize it effectively. A data analytics framework is critical in organizing, analyzing and making sense of this information – turning numbers into actionable insights you can act upon. This guide will introduce various data analysis frameworks and how they can be effectively employed.
Table of Contents
What is a Data Analytics Framework?
Data analytics frameworks are defined as methodologies or structured approaches that facilitate the interpretation and analysis of data to gain meaningful insights and make informed decisions. A data analytics framework serves as a structured approach or methodology which guides organizations in using consistent processes for data analysis. A framework acts as a guide, helping ensure organizations adhere to a consistent data analysis process.
Data analytics frameworks may evolve as technology evolves and new analytic techniques emerge, so organizations must constantly evaluate and update their frameworks to stay on the cutting edge of data analysis.
Components Of a Data Analytics Framework
A data analytics framework serves as the backbone of data analysis. It ensures everything goes smoothly from beginning to end and contains steps designed to help you comprehend large volumes of information. Let’s take a closer look at its main components.
Data Collection and Acquisition.
Data collection is the foundation of any project that relies on data. It involves gathering information from different sources, such as databases, sensors, and online interactions, with the goal of ensuring the data gathered is high-quality and pertinent to solving the issue at hand.
Data Cleaning And Processing
Once acquired, data is rarely in an easy-to-analyze form. It often contains errors, redundant information, or irrelevant facts. Therefore, data must be cleaned and processed to make it usable before being refined using techniques such as data wrangling and normalization.
Data Storage
With big data, managing vast amounts of information efficiently requires effective storage methods. Databases, data warehouses, or cloud solutions may all be appropriate options, depending on your analysis requirements and the scale of the information.
Data Analysis and Mining
Once your data is clean, the next step should be extracting patterns and insights. Data analysts use statistical tools, machine-learning algorithms, or even simple Excel functions to delve deep into datasets in search of correlations, patterns or anomalies that require further examination.
Visualizing data can significantly facilitate comprehension and interpretation. Tools like Tableau, Power BI or Python libraries like Matplotlib can quickly turn complex datasets into easily understandable charts, graphs or dashboards for stakeholders to interpret and access.
A Step in the Decision-Making Process
The framework’s primary goal is to enhance business decisions. Once insights from visualizations and analyses have been gleaned, they are shared with decision-makers for consideration and action to increase efficiency or growth can be taken accordingly. This component seeks to achieve this end.
Types of Data Analytics
Descriptive analytics employ past data to describe and explain what has occurred, providing insight into why current business performance is as it is. Data visualization, business reporting, and dashboards are common techniques employed here.
Diagnostic Analytics: Diagnostic analytics seeks to understand what has occurred by correlating and examining patterns found within data to detect organizational problems or opportunities. Data mining and drilling down techniques may also be employed.
Predictive analytics: utilizes statistical models and forecasting techniques to predict future outcomes. Current and historical data are used to make predictions; regression analysis and machine learning are two such techniques.
Prescriptive analytics: Prescriptive analytics is an analytical approach that offers advice based on a predictive model to achieve goals more quickly and cost-effectively. Techniques used include simulation, optimization, and decision modelling.
Conclusion
Data analytics can be an intricate journey. However, with the appropriate tools and a solid framework for data analysis, businesses can streamline their approach to data-driven decision-making. A framework forms the cornerstone of an effective analytics strategy, helping companies understand past trends using descriptive analytics, forecast future outcomes using predictive models, make real-time decisions, and take organizational needs and each framework’s strengths and weaknesses to make an informed decision when selecting one or more suitable options.
Also Read : Advanced Data Analytics: Techniques And Applications