Advanced Data Analytics: Techniques And Applications
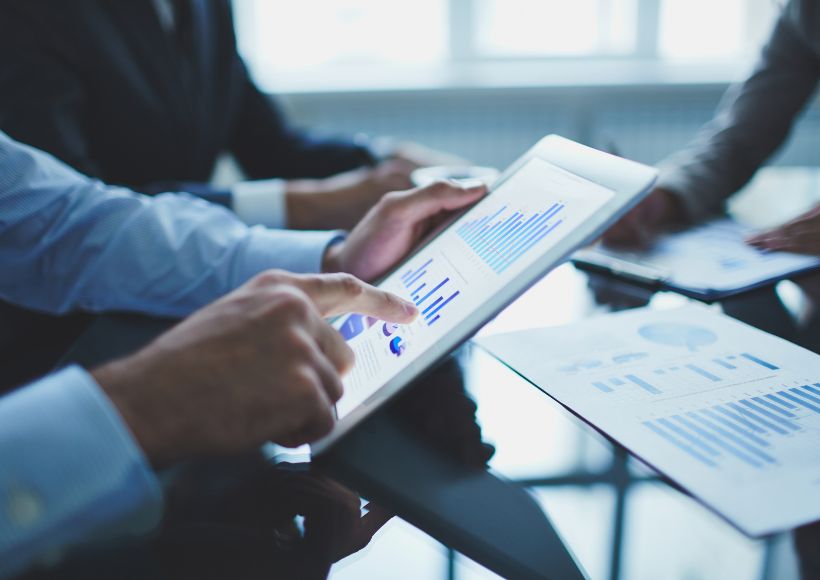
Data analytics has even gained a significant position in the contemporary business environment. Due to the constant surge in data, organizations are adapting advanced measures to extract insights and make decisions.
Let’s get into some of the most popular Advanced Data Analytics Techniques that are new in the field and some business applications. It will assist you in opting for a certificate in Business Analytics which will impart knowledge regarding business data analysis.
Table of Contents
Cohort Analysis
Cohort analysis is one of the most important tools for designing an optimal promotional or advertising campaign at the appropriate time. It assists in identifying the right time to send reminders to the customers so as to elicit the most interest.
When utilized effectively, cohort analysis offers businesses the following advantages:
The first is that by improving retention, cohort analysis improves the total revenue obtained from the customers over the whole time that the clients are with the business.
Loyal clients can be spotted, and cohort analysis provides reasons that allows the business to encourage these clients to remain with them.
Cohort analysis easily provides an answer to the question of how to evaluate new products under development, when the goal is to situate new designs in such cohorts and compare their conversion rates with those that did not participate.
Predictive Analysis
Predictive analysis uses data mining, machine learning, and statistical models to accurately predict future events. It has gained popularity because it can provide valuable insights for business planning.
Here’s how predictive analysis enhances business performance:
Predictive analysis helps anticipate consumer responses to product changes, enabling businesses to attract and retain customers effectively.
Predictive analysis improves operational efficiency and reduces costs by accurately managing resources like inventory and staffing.
Behavioral predictive analytics identifies anomalies and patterns in real-time, aiding in fraud detection and addressing potential security threats.
Predictive analysis assesses a buyer’s creditworthiness, predicting their payment reliability and reducing business financial risks.
Cluster Analysis
Cluster analysis is the classification of data through grouping phenomena according to their likenesses and those outside these groupings. Its uses are most common in marketing, insurance, and geology.
Cluster analysis consists of three main types.
The Hierarchical Cluster approaches enables the creation of numerous subsets from an existing core containing all attributes to smaller units which can be formed over a specific period which makes analysis of various datasets much easier than before.
K-means algorithms can effectively clasp large data sets into one cluster. Ther estimates an initial number of clustering and compares the various models accordingly.
Two-step Clustering make use of both K-means and hierarchical cluster analysis procedures. It begins with a pre-clustering stage that employs clustering algorithms and only then is hierarchical cluster analysis approaches used. This is helpful in situations where conventional hierarchical analysis approaches are inconducive to large datasets.
Machine Learning
Although machine learning is an intimidating term, it does in fact simply refer to computational techniques to discover patterns in data and construct statistical models for further data sets.
There are different kinds of machine learning
Supervised learning: Here, machines analyze vast quantities of unlabeled information, derive useful patterns, and are able to make favorable predictions.
Unsupervised Learning: In this case, algorithms automatically assemble data based on its given likenesses and relations and do so without being prescripted goals or objectives.
This method of learning utilizes both labeled data and unlabelled data which is then used to train the model while any remaining information is labeled afterwards.
Reinforcement Learning: This method, which is relatively new, attempts to find a solution through trial and error while receiving rewards and penalties from reaching that solution.
Complex Event Analysis
Complex Event Analysis or Complex Event Processing (CEP) on the other hand involves causality inference that analyzes multiple events and identifies their relationships. The practice of targeting consumers who can/will only stick around for a complex event is commonplace and quite successful when completed with a low and controllable lag.
Other Common Applications Are
Using complex event analysis for behavioral marketing, marketers can identify customer’s behaviors and provide targeted promotions. Likewise, using the algorithms makes it easy for marketers to determine the trends in stock prices to help them sell or purchase at the correct time.
Thus improving production quality, manufacturers are able to utilize Complex Event Analysis for monitoring information and predicting operational trend indicators for the components.
When provided with smooth and seamless data feed, activation of CEP capabilities helps in elevating automated vehicle systems such as aware of existent road signs, distance, and braking adequately.
Using these techniques effectively can provide significant benefits in the areas of business, such as: marketing, finance, and operations. Utilizing their power will assist companies in making decisions that are backed with data which will aid their business in this complicated age.
In today’s environment, everything is so fast and focused on data, that data analytics has become a necessity. This is a good time for anyone wanting to enter this field or those who are simply interested and want to know more about it. Such certificate programs are available to give this training – perhaps you need one?
Business Analytics vs. Data Analytics – Difference Explained